10 minute read
Knowledge graph visualization: A comprehensive guide [with examples]
Learn how knowledge graph visualization provides a clear understanding of data relationships through its challenges, benefits, applications & tools.
Table of contents
Knowledge graph visualizations are powerful business intelligence tools that offer a visual representation of complex relationships and connections within vast amounts of data.
Knowledge graph visualization provides your business with a clear and intuitive means of understanding and exploring intricate networks of data points.
By presenting information in an organized, visually appealing and interactive manner, these visualizations enable decision-makers to gain valuable insights, identify patterns, and make well-informed strategic choices.
From identifying customer behavior patterns to optimizing supply chain management, knowledge graph visualizations play a crucial role in solving a wide range of business challenges, empowering organizations to unlock the true potential of their data.
Let's take a closer look at knowledge graph visualization, its different charts, uses, challenges, benefits, and tool types.
What is knowledge graph visualization?
Knowledge graph visualization is the process in which a knowledge graph is depicted in a visual format. It shows how data is connected in a way that's easy to understand and search.
A knowledge graph, or semantic network, is the medium used to display real-world concepts the data represents (nodes) and how they are connected (edges).
A knowledge graph acts as a database. The knowledge it displays is portrayed as an interrelated set of edges and nodes, each symbolizing an entity.
The edges symbolize a connection between two existing entities. The graphs display how these edges and nodes interact. This allows the viewer to understand how they're related more readily.
Think of a family tree. Each person (node) is represented, and their relationships to each other (edges) are clearly mapped out. The same happens in a knowledge graph:
Knowledge graphs can incorporate pretty much anything you could possibly have data on, including:
- People
- Places
- Objects
- Events
- Situations
- And even concepts.
The relationships represented can be as simple or complex as you need them to be.
They can be tailored to your purpose to create the ideal knowledge graph visualization for your data set. This flexibility makes them useful for a wide variety of situations.
Knowledge graph visualization serves as a storytelling tool.
Your data exists but not in a vacuum.
Using a knowledge graph visualization involves putting that data in its proper context and telling a more holistic, comprehensive story of what it means for your business.
How do you visualize knowledge with graphs?
Creating knowledge graph visualizations requires a series of steps starting from identifying and collecting the entities you want to capture as part of this visualization.
1) Data retrieval and selection
- Collection: Gather all structured and unstructured data from all databases and other storage locations.
- Streamline: Remove any data that isn't relevant, including duplicate information.
- Extraction: Extract entities and relationships from the data.
2) Graph building
- Construct: Use a graph database and the appropriate tooling to construct the knowledge graph. (A list of the top tools is provided later in this blog post).
- Populate: Insert entity and relationship information in your knowledge graph.
Choose and build the chart
When it comes to constructing your knowledge graph, you also have to decide how you want to visualize and build a chart considering factors such as the nature of the data, intended audience, and insights you want to convey. (In order to build a chart, you have to extract and transform data from the knowledge graph).
There are several techniques and chart types that can be employed to represent the intricate relationships and hierarchies within the data effectively. Here are the most common ones:
- NODE-LINK DIAGRAMS
One common approach is the use of node-link diagrams, which display the graph as a network of nodes connected by edges. These diagrams are most effective when visualizing knowledge graphs with complex relationships, targeting a diverse audience (technical and non-technical, e.g. business executives or decision-makers), and aiming to extract insights related to the graph's structure, connectivity, and patterns (e.g., clusters, communities).
A visualization of Jürgen Röhm social network, based on his Facebook friends in early 2013.
Source: Jürgen Röhm - MATRIX-BASED VISUALIZATION
Another relevant technique is matrix-based visualization, where the graph is represented as a matrix, with rows and columns representing entities and intersections indicating relationships between them. They are well-suited for tabular or hierarchical data and intended for audiences with varying technical backgrounds (technical or non-technical, e.g. business analysts or executives). They provide insights into the relationships, strengths, and patterns within the graph.Source: Lucidworks
- TREEMAPS AND SUNBURST
Treemaps and sunburst charts are also valuable for visualizing hierarchical structures within the knowledge graph. These techniques help to uncover patterns, clusters, and outliers within the data, enabling businesses to gain deeper insights and make data-driven decisions. These charts are suitable for knowledge graphs with hierarchical or nested data structures, targeting audiences of both technical and non-technical stakeholders. They offer insights into the distribution, proportions, and relationships within the graph.
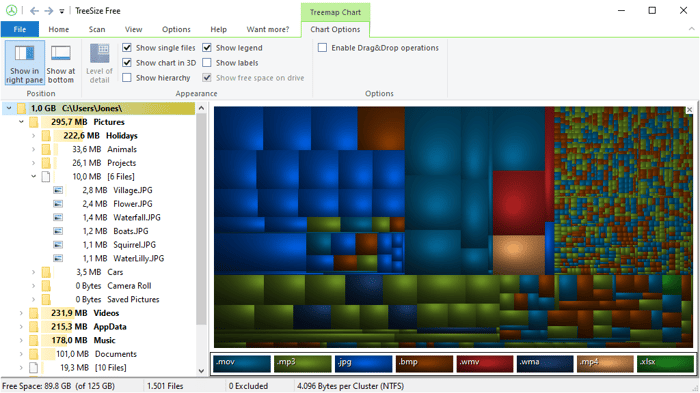
The hierarchical treemap chart shows a colored square for each folder, file type, or available space on a drive.
Source: Jam Software
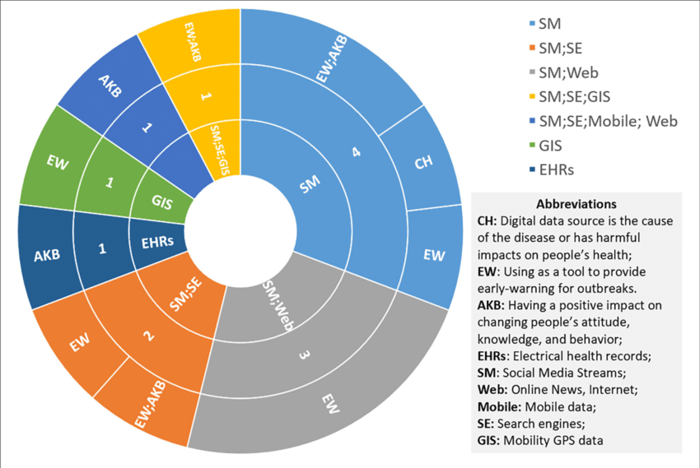
Sunburst chart displaying the impact of each digital source on health emergencies.
Source: ResearchGate
3) Graph calculations
Various metrics or indicators can be computed for the graph to enhance its visualization.
4) Graph layout
Graph layout involves determining the spatial arrangement of vertices in order to create a visually appealing representation and make it easier for human users to understand the underlying information.
5) Rendering
Ultimately, the graph is presented or rendered on the screen for viewing.
At this point, you have the opportunity to analyze your data. You can filter the data, categorize and sort, and draw conclusions from what you see. You can use this visualization to present data-backed stories.
But you have to remember that the knowledge graph must be maintained, updated, and monitored!
Uses of knowledge graph visualization
Knowledge graph visualizations can be found across every industry, from retail to health care, and they can serve many different purposes.
Thanks to the flexible and customizable nature of knowledge graphs, their forms and functions are as diverse as databases and data sets are. Let's go over some examples that best illustrate the concept and some of the most popular business use cases.
Search engines
One of the most helpful and universally familiar examples is the kind of knowledge graph used by search engines.
When you search for anything, the goal is to deliver you the most relevant information possible. To do this, search engines use knowledge graphs.
For example, if you search for "Harrison Ford", you'll get results that relate directly to him, like his photos, his Wikipedia about section snippet, and the most recent news stories about him.
These are all helpful if you want to learn specifics about him, find the latest gossip, or remind yourself what he looks like.
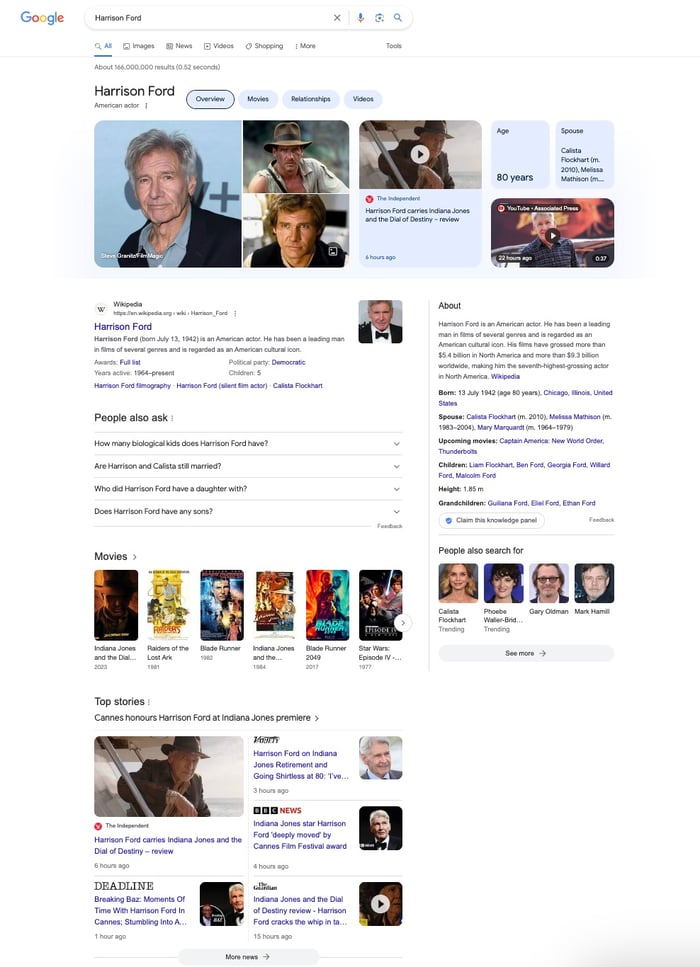
Source: Google
However, people often search for actors' names when they're thinking of a film that they can't remember the name of but know who was in it. That's why they provide a section listing all his films that you click on to search for more information on them.
Source: ahrefs
Search algorithms use knowledge graphs to understand how all these topics are connected. This allows them to provide you with these specific and helpful results.
Search algorithms are responsible for helpful sections like:
- Related topics
- People also asked
- The tags at the top of the page to help you refine your search
Advertising strategies
Advertising is another example of a use case for knowledge graph visualizations. These use of knowledge graphs visualizations give a 360-degree view of the interactions a customer has – for example - with the company's product.
Many companies are, in fact, able to collect a variety of data and examine it. For example, customer touchpoints, products they liked or rated, participation in loyalty programs or promotions.
Source: Data Geeks
With this information, companies can study their customers’ behaviors and gain insights. They can uncover correlations between touchpoints and purchasing decisions, identify customer segments with distinct preferences, and make data-driven decisions to optimize their advertising strategies.
For example, if the visualization reveals that customers who participate in specific partner programs tend to have higher loyalty card scores and make more frequent purchases, the company can allocate resources toward promoting and expanding those programs.
Source: Mirror
They can personalize advertising campaigns to target customers based on their preferences, tailoring messages and offers to increase engagement and drive conversions.
Website content searchability
Knowledge graph visualization is great for both large and small businesses. It can be used to create a helpful knowledge base to make your content searchable and make your on-site experience better for users.
User experience metrics also help your site rank high on Google, lead to return customers, and contribute to higher satisfaction levels.
You could use a knowledge graph on your website to:
- Help customers search for products
Source: Ontotext - Help them navigate your help pages
Source: Medium
Product recommendations
Returning customers are the most profitable part of any business. They're much easier to convert, and they tend to spend more than new customers.
The key to effectively leveraging your existing customers is to provide them with a great experience they want to repeat and keep in touch with new product release information, deals, and other helpful communications.
The mechanism behind this process is like the one happening for movie recommendations in some streaming services:
Source: Medium
Likewise, retail companies send customers product recommendations based on demographic information and purchase history.
For example, if you know they're a parent, you can recommend toys. If you know they bought a changing table from you, recommend the matching crib and dresser.
Source: Amazon
Challenges and implementation frameworks
The biggest challenge in knowledge graph visualization is taking all the random, unstructured data from its various data storage mediums and silos and converting it into structured, centralized, usable information.
Data is information without context. While you have no problem looking at various kinds of data and understanding the context that makes it useful, computers have no such ability.
(Which is why humans build algorithms to tell them what to do)
Take a list like this, for example:
You can look at that list and know that one is a video platform, one is a cereal brand, and one is a car manufacturer. A computer just sees random words without that context.
Metadata, or information about data, provides the context required for computers to understand that. This is done via ontologies that describe categories, nodes, and relationships.
With the proper framework (e.g., Extensible Markup Language (XML) used on websites and Resource Description Framework (RDF) used by business solutions) computers can understand what data means, what it's about, and how it's connected.
This semantic layer makes it possible to search for information more easily because the system understands what you're asking for.
Even when systems use different terms to label the same things, a comprehensive semantic network can understand that these are synonyms and provide the complete data set contained within the concept and all its descriptors.
Benefits of knowledge graphs visualization
The visualization of knowledge graphs offers several benefits that enhance understanding, exploration, and analysis of complex information networks.
Here are some benefits of knowledge graph visualization.
Improved comprehension
Knowledge graphs often contain vast amounts of interconnected data, making it challenging to grasp their structure and relationships.
Visualization techniques make it easier to understand complex information by presenting it in a visually intuitive and accessible manner. Users can quickly identify patterns, clusters, and hierarchies, leading to a deeper comprehension of the underlying data.
Enhanced exploration and navigation
Visual representations of knowledge graphs allow users to explore and navigate the data more effectively. Through interactive interfaces, users can interact with the graph, expand or collapse nodes, zoom in or out, and navigate through different levels of abstraction.
This flexibility enables users to uncover hidden connections, explore different paths, and gain insights that might not be immediately apparent in a textual or tabular representation.
Facilitated knowledge discovery
Visualization aids in knowledge discovery by highlighting relationships and connections between entities. By visualizing the graph, users can identify clusters of related information, detect outliers, and reveal previously unknown associations.
This can lead to the discovery of valuable insights, correlations, or patterns that might otherwise go unnoticed.
Support for decision-making
Visualizing knowledge graphs can assist in decision-making processes. By presenting data in a visual form, decision-makers can better comprehend complex scenarios, assess the impact of potential decisions, and identify potential risks or opportunities.
Visualization enables a more holistic and intuitive understanding of the information, enabling more informed and confident decision-making.
Effective communication
Knowledge graph visualization is an effective mean of communicating complex information to diverse audiences. Visual representations can simplify the presentation of intricate concepts and relationships, making it easier for non-experts to grasp the content.
Visualization facilitates effective communication and knowledge sharing among different stakeholders, enabling collaboration and fostering a shared understanding of the information.
Integration of diverse data sources
Knowledge graphs often integrate data from various sources and domains. Visualization allows users to see the connections between these disparate data sources, enabling them to explore relationships that span multiple domains.
This integration can provide a broader perspective and enable cross-domain analysis, leading to new insights and discoveries.
Types of knowledge graph visualization tools
Many tools can aid you in the creation of your knowledge graph visualizations.
Here are some of the most popular tools available:
Databases: There are many databases available to help you store your information. The right database will vary by business and use case.
An example are RDF databases and OWL language.
- RDF databases are specialized databases that store and manage data using the Resource Description Framework (RDF) model. RDF represents information in the form of subject-predicate-object triples, enabling flexible and scalable data integration and querying.
- OWL (Web Ontology Language) is a semantic web language that allows the creation and sharing of ontologies, which are formal representations of knowledge domains. OWL provides a rich vocabulary for expressing complex relationships and logical constraints, enabling advanced reasoning and inference capabilities for knowledge-based systems.
Together they form a powerful combination for managing and reasoning with structured data, facilitating semantic interoperability and intelligent information processing in various domains.
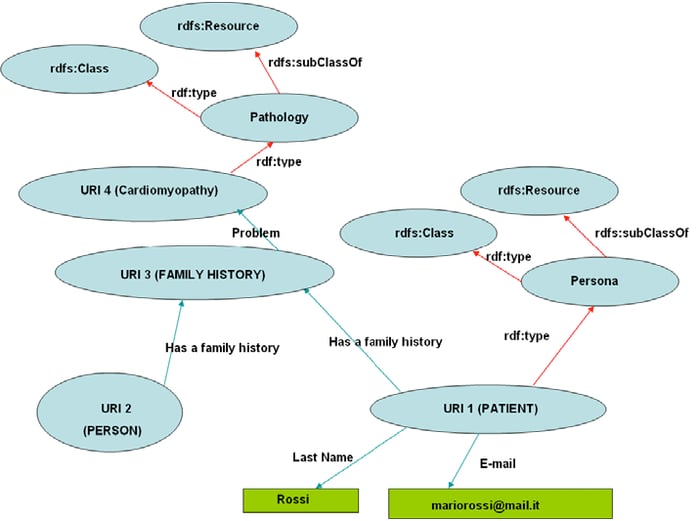
Source: Research Gate
Data intelligence platform: A platform like Datavid Rover allows you to connect your data, extract information, and conduct fast, easy searches.
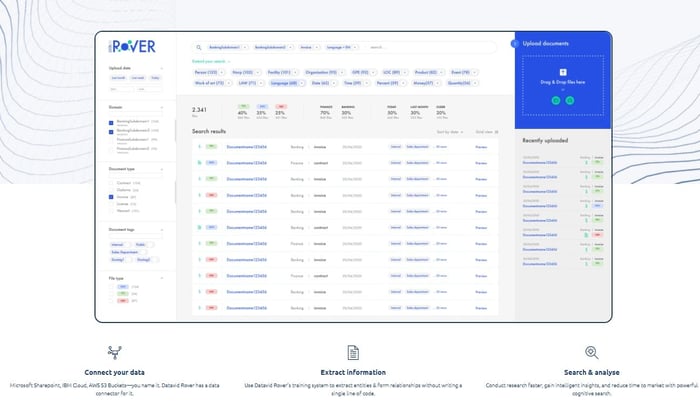
- Language model: A natural language processing (NLP) model like GPT-3 can help your system understand things like search queries.
Source: Chat GPT
Machine learning platform: A machine learning platform like Google's TensorFlow can help you analyze your unstructured data.
Source: Google TensorFlow
Find the right knowledge graph visualization tools
There are several knowledge graph visualization tools from different knowledge graph companies available that can help in visualizing and exploring knowledge graphs.
These three tools are well-regarded for their capabilities in visualizing knowledge graphs, each with its own strengths and features. The choice among them depends on specific requirements, such as data format, scalability, customization needs, and integration capabilities.
Cytoscape
Source: Cytoscape
Cytoscape is a versatile and widely-used open-source tool for visualizing and analyzing complex networks, including knowledge graphs. It offers a range of layout algorithms, styling options, and interactive features to create visually appealing and interactive visualizations. Cytoscape also provides various plugins and extensions for advanced analysis and customization.
Gephi
Source: Gephi
Gephi is an open-source graph visualization and exploration tool that supports various graph formats, making it suitable for visualizing knowledge graphs. It offers a user-friendly interface with a wide range of layout algorithms, filtering options, and interactive visualization features. Gephi is particularly known for its ability to handle large-scale networks efficiently.
KeyLines
Source: Dataversity
KeyLines is a commercial JavaScript toolkit for building interactive and visually appealing graph visualizations. It provides a range of features like zooming, filtering, and dynamic querying to explore and interact with knowledge graphs effectively. KeyLines offers customization options and supports integration with various back-end technologies.
Other knowledge graph visualization tools
Neo4j Bloom
Neo4j Bloom is a graph visualization and exploration tool specifically designed for Neo4j, a popular graph database. It provides an intuitive interface to navigate and explore knowledge graphs stored in Neo4j visually.
Ontotext GraphDB
Ontotext GraphDB is a semantic graph database that supports the RDF data model and offers scalable storage and querying for knowledge graphs. It provides features like semantic search, reasoning, and integration with various text-mining tools.
Stardog
Stardog is not specifically a knowledge graph visualization tool; instead, it is a leading enterprise-grade knowledge graph platform that enables the creation, management, and querying of knowledge graphs. It provides robust features for data integration, ontology modeling, reasoning, and powerful query capabilities using SPARQL.
Frequently asked questions
Knowledge graph visualization is the representation of data and relationships in a visual format to gain insights and understand complex information.
To draw a knowledge graph, you typically use specialized graph visualization tools or libraries that allow you to define nodes and edges to represent entities and relationships, respectively.
There are several excellent tools available for creating knowledge graphs, including Cytoscape, Gephi and KeyLines,but also Neo4j.