3 minute read
Data discovery process: 5-step guide to extracting new insights
The data discovery process makes data meaningful by finding hidden patterns and trends. Here is a 5-step guide on how it works.
Table of contents
Data discovery is a process, not a tool or technology. It’s an iterative process of drawing out insights by finding latent patterns and outliers, enabling a better understanding of organisational data.
Deciphering the meaning of data to gain a competitive edge requires an efficient data discovery process.
Companies now generate over 2.5 billion gigabytes of data every day.
Hard to process, right?
Out of this, 70% is user-created data.
(Source: Exploding topics)
Why am I telling you these facts?
This volume of data requires efficiency in data collection, discovery, and analysis. But let me start from the beginning…
What is data discovery?
Data discovery is the process of collecting and processing data available throughout the organisation to understand trends, patterns, and relationships. Note the emphasis on the latter.
The process is iterative in nature and provides a 360-degree view of the entire business to make decisions and support business goals.
5 steps for a great data discovery process
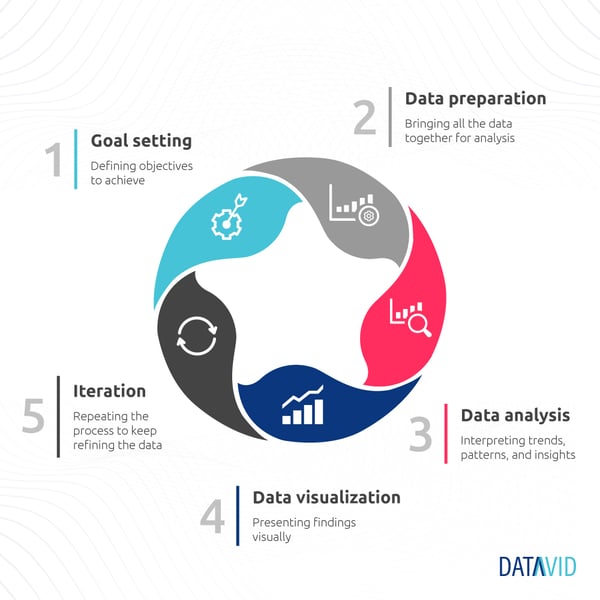
The whole process is iterative in nature and provides you with insights and visual mapping to clarify the data your enterprise holds.
Let’s take a look at the key steps in data discovery.
Data discovery step #1: Goal setting
Start by answering these questions:
- Why do we need a data discovery process?
- What is the business objective we’re trying to achieve by implementing one?
Business objectives should be broad at first.
For instance, an objective could be:
“We want to understand more about our users’ interactions with our products.”
Another example:
“We’re looking at ways to achieve higher regulatory compliance.”
Clarifying what you set out to achieve will help you remain focused.
Data discovery step #2: Data preparation
Data preparation starts with bringing all data generated through various sources—external or internal—to one place where it can be accessed and analysed.
Combining the relevant data is achieved by data integration and ingestion.
Data residing in disparate sources is brought together, cleansed for errors, formatted in a standardised manner, and transformed to provide a complete picture.
Data discovery step #3: Data analysis and developing insights
Integrated, cleansed data sets the stage for data analysis. At this stage, data is analysed to interpret patterns and uncover hidden insights.
The analysis largely depends on the objective identified in Step 1.
Data discovery step #4: Data visualisation
The entire analysis and the insights extracted are shared with business users in visual forms, such as dashboards, graphs, charts, maps, etc.
This enables easy processing of the outcome generated by a massive amount of data. Decisions are quicker as you can focus on specific business objectives.
Data discovery step #5: Iteration
Solving business problems through data discovery is an iterative process.
With information ever-changing, the data discovery process has to be repeated to remain relevant, as business goals are subject to change over time.
Benefits of data discovery
Data in any organisation resides in various units and sources, leading to “data drowning”—essentially getting lost around rapidly growing volumes of data.
Having a good data discovery solution helps in having a good grip on your information by:
- Ensuring data quality: Accuracy, completeness, standard, and consistent format.
- Data-driven intelligence: Strategic decisions by collecting existing data methodically, analysing it, and extracting meaningful insights.
- Enhanced regulatory compliance: Like GDPR and HIPPA, this involves safeguarding confidential information by hiding sensitive, personally identifiable or personal health information from unauthorised personnel around the organisation.
- Reducing vulnerability: Tracking down the location of sensitive information across the enterprise to prevent data leaks from internal and external actors.
I’ve covered the 5 core steps to help you start with the data discovery process: 1) Goals, 2) Preparation, 3) Analysis, 4) Visualisation, and 5) Iteration.
Datavid makes this process simpler by introducing Datavid Rover, the knowledge engine for enterprises that want to increase data compliance and lower costs.
It gains over traditional platforms by identifying occurrences and trends, uncovering relationships, and visualising real-world entities in a knowledge graph.
This article will clarify the data discovery process to get started with taking control of your data.
Frequently Asked Questions
Why do we need data discovery?
Data discovery helps in revealing the patterns, trends, and relationships within the data which remain concealed otherwise.
What are the steps in data discovery?
The steps in data discovery are 1. Goal Setting, 2.Data preparation, 3.Data analysis, 4.Data visualization, and 5.Iteration.
How does data discovery result in data-driven intelligence?
In data discovery, data is collected methodically and analysed through visual tools to reach meaningful conclusions.