3 minute read
Why data integrity is important for your enterprise [2023]
The more complex a system, the higher the chances for data quality to degrade. Learn why data integrity is so important for your enterprise.
Table of contents
“Data” isn’t just a generic word. It represents the smallest unit of value that your enterprise produces, and its integrity is the key to scaling that value to customers globally.
That’s because integrity is all about ensuring that your data does not:
- Become less accurate over time
- Lose meaning and, therefore, value
- Decrease in quality as systems evolve
- Lead to potential compliance issues
- Compromise your brand’s IP
The benefits of good data integrity are clear even at a small scale but are especially (and crucially) evident at a large scale. Think life sciences enterprise with a lot of IP to protect and constant regulatory scrutiny—the only way to satisfy both is through good data integrity.
Let’s explore why.
What is data integrity? A quick refresher
Data integrity is a set of data management, processing, storing, and access practices that come together to ensure the accuracy and consistency of data over time.
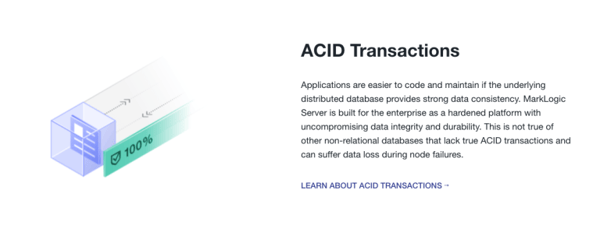
On small projects, giving integrity less thought might seem like an acceptable practice, but it almost immediately becomes an issue when the system reaches any level of scale.
Many enterprise businesses follow old, rigid ways of managing data, wasting a lot of time manually piecing it together and losing meaning in the process.
The big problem with large-scale data integrity
For many years—and still today—dealing with data integrity in large enterprises meant implementing ETL (Extract-Transform-Load) processes that required extensive human input.
This worked well and still works for mid-market companies with steady and somewhat predictable rates of change. The big problem is when a company cannot afford to change linearly.
“ETL works well at somewhat predictable rates of change. But it breaks down when a company cannot afford to change linearly.”
In 2023, large-scale enterprises had to deal with a constant barrage of lean technology startups claiming to be the “next big thing” in their respective fields of expertise.
These are real threats to existing businesses, and they come with the weight of having to compete with agile teams that spend all of their time improving data processes with none of the historical baggage.
How to scale services without jeopardising data integrity
Because of the startup threat, enterprises are forced to adopt more automated, efficient, and ultimately more complex data management processes.
The risk with this endeavour is high:
- Legacy data is often incompatible with new technologies
- The knowledge required is deeply-technical & very specific
- Failure means incurring both financial and reputational setbacks
There is a lot at stake, and unlike small startups, enterprise companies need to deal with putting 1000s of employees first—not the technologies or the processes.
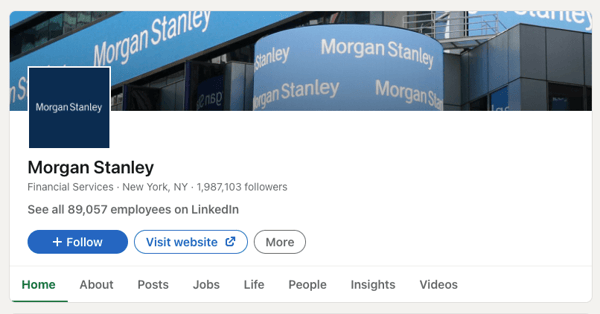
To achieve great data integrity standards at high rates of change without putting other business assets at risk, your enterprise should invest in 2 key areas:
The former gives you insight into your data so you can make informed decisions about how to enrich your services and the integrity pitfalls to avoid when scaling them.
The latter is a way of rapidly testing and bringing solutions to the market for end-users to get their hands on. If things don’t work out, pivoting is simple and even encouraged.
“Unlike small startups, enterprise companies need to deal with putting 1000s of employees first—not the technologies or processes.”
But if they do work, you’re effectively beating the competition while considering the decades of insights only your enterprise has when compared to a months-old startup.
Making the most of your data in 2023 & beyond
Enterprises in industries like life sciences, financial services, and publishing are all experiencing wide, horizontal shifts in consumer and business behaviour.
Without proper data integrity, your enterprise is prone to risks such as losing existing business, failing to prove data origin for compliance audits, and leaking IP.
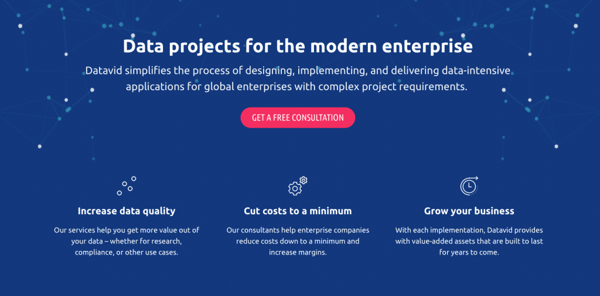
Consulting firms like Datavid are committed to helping enterprises solve the big puzzle of data intelligence, including integration, automated processing, security, and integrity.
All of this is at scale.
With intelligence at the centre of your data strategy, your enterprise can extract and visualise valuable knowledge and use it to enrich high-growth services without jeopardising integrity.
If you want to achieve data integrity at the highest standards, consider scheduling a free 30-minute call with a data expert today and beat the competition to its own game.
Frequently Asked Questions
What is data integrity?
Data integrity refers to the accuracy, consistency, and reliability of data throughout its lifecycle. It ensures that data remains intact and trustworthy, free from errors, inconsistencies, or unauthorised modifications. Data integrity is crucial to maintain the quality and reliability of data for effective decision-making, analysis, and operations.
What is an example of data integrity?
An example of data integrity is ensuring that a database or dataset is accurate, consistent, and reliable. For instance, if a database contains customer information, data integrity would mean that the information is complete, up-to-date, and without any errors or inconsistencies, such as duplicate or missing records.
What are the four components of data integrity?
The four components of data integrity are accuracy, completeness, consistency, and reliability.