6 minute read
Exploring semantic AI: Definition and 10 business benefits
Maximize the business value of your data. Learn how semantic AI uses Machine Learning and Natural Language Processing to help you succeed.
Table of contents
One of your company's greatest assets is the data you accumulate.
But how do you maximize the business value of that information?
One of the newest and most rapidly growing branches of artificial intelligence, called semantic AI, aims to help you do exactly that.
What is semantic AI?
Semantic AI combines machine learning (ML) and natural language processing (NLP) to enable software to comprehend speech or text at a human-like level.
It considers not only the meaning of the words in its source material but context and user intent as well.
Information resources within most organizations exist as isolated and disconnected data stores that cannot be easily integrated.
Reasons for this include:
- Data is housed in different locations or systems and is not consolidated or accessible through a single interface.
- Data is stored in a variety of formats, both structured (spreadsheets, for example) and unstructured (text documents, emails, etc.).
- The logical relationships between data items is not clear or direct.
When your data is fragmented this way, it can be difficult to comprehend how it all fits together and extract the information you need from it.
Semantic AI and data platforms consolidate all your sources and types of data into a single logically unified, searchable, and comprehensible knowledge pool.
It identifies logical relationships within the data, enabling you to glean valuable business insights and use that information to solve real-world problems.
How does semantic AI work?
Semantic AI's understanding of context enables it to comprehend the meaning of its data and the intent of users.
ML algorithms are limited in their ability to understand meaning because they analyse data in isolation, taking no account of context (how one fact relates to others). This is a limitation.
For example, a machine learning algorithm of online stories is unable to understand that wool is warm and that is why people wear it in winter.
NLP has similar issues. It's now very good at understanding human language the way people actually use it.
For example, if you've ever tried to carry on an extended conversation with a smart speaker, such as Amazon's Echo or Apple's Siri, you've probably been frustrated by NLP's limitations in comprehending context and user intent.
Semantic AI overcomes these limitations by adding a crucial third element to the foundation provided by ML and NLP: the knowledge graph.
Semantic AI and knowledge graphs
According to IBM, knowledge graphs visually represent the relationships between real-world entities, such as objects, events, situations, or concepts.
By exposing the relationships between entities, knowledge graphs enable semantic AI applications to manage and present information based on contextual meaning and logical correlations.
Benefits of semantic AI
Machine learning is one of the most widely used technologies for building AI systems. But semantic AI provides solutions that ML alone cannot.
To maximize the value of your organization's data resources, semantic AI is a game changer.
Here are some ways your business can benefit from it:
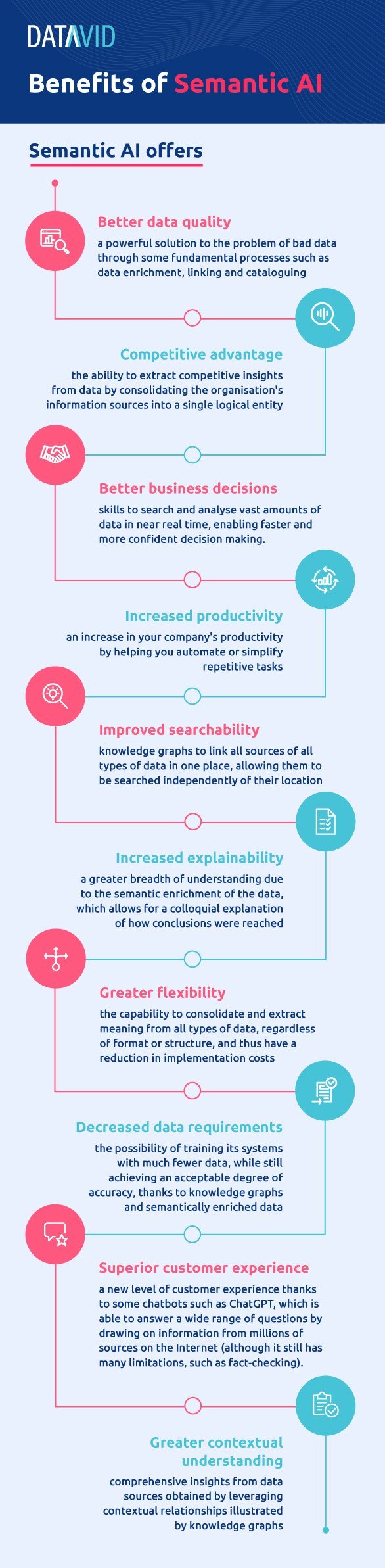
#1 Better data quality
Data is the lifeblood of modern businesses. But, as a recent Forbes warns, low-quality data is a widespread issue that's undermining the success of many businesses today.
If you want to minimize this issue for your company, you'll need to institute a data governance strategy that effectively oversees and manages your data throughout its entire lifecycle.
Semantic AI, because of the data enrichment, linking, and cataloguing that are fundamental to its processes, provides a powerful solution for the bad data problem.
Semantic AI addresses the need for interpretable and meaningful data, and provides technologies to create this kind of data from the very beginning of a data lifecycle.
#2 Competitive advantage
By consolidating your organization's information sources into a single logical entity, semantic AI enables you to extract deeper insights from your data.
For example, semantic AI's sophisticated semantic analysis abilities can make you earn valuable insights from customer feedback, providing a comprehensive understanding of how customers feel about your products or services, and where you could improve.
That kind of information can be invaluable in helping you outpace your competition by better meeting customers' expectations and needs.
#3 Better business decisions
Because of its ability to search out and analyze vast amounts of data in near real time, semantic AI can provide new insights that, for example, help you to streamline your company's internal operations or recognize marketplace trends and opportunities you may have overlooked.
That, in turn, allows you to make better business decisions more quickly and confidently.
#4 Increased productivity
Semantic AI can boost productivity in your business by helping you automate or streamline repetitious tasks.
For example, low-code/no-code (LCNC) platforms are now widely used to allow non-technical workers to automate portions of their workflows.
Semantic AI, which has been also called "LCNC on steroids", can make it even easier for your workers to translate natural language instructions into workable software.
#5 Improved searchability
Semantic AI uses knowledge graphs to link all your data sources (both structured and unstructured) together, allowing databases, documents, spreadsheets, emails, etc., to all be viewed as a single logical entity that can be searched for information regardless of its location.
#6 Increased explainability
The most pressing issue with traditional ML is its lack of explainability. Most modern ML algorithms use neural networks. Although such networks can produce good answers based on their input data, they appear to users as "black boxes" where only the inputs and outputs can be seen. The factors that produced a particular answer are unknowable.
That is because ML algorithms don't work by reasoning based on facts extracted from their training data. Rather, the system is trained by adjusting weighting factors at the nodes of its neural network. And those weights, though observable, don't correspond to anything in the real world.
Instead, the greater breadth of understanding imparted by the semantic enrichment of its data allows a semantic AI system to conversationally explain how it reached its conclusions.
That has huge practical and legal implications. A system that produces seemingly accurate conclusions but can't explain them cannot be held accountable, and people won't trust it. And what people don't trust, they'll ultimately refuse to use.
#7 Greater flexibility
Traditional ML models are typically customized for each use case because there's no standard ML architecture that performs equally well in all situations. For example, most ML algorithms can work with either structured or unstructured data but can't combine the two.
In contrast, semantic AI can consolidate and extract meaning from all types of data, whatever the format or structure. Because of that flexibility, semantic AI implementations don't have to be customized for each use case, which translates directly into lower costs.
#8 Decreased data requirements
To get accurate results, traditional ML requires huge amounts (often millions of records) of training data. Obtaining such massive datasets for your specific use cases can be difficult, time-consuming, and expensive.
On the other hand, the use of knowledge graphs and semantically enriched data allows semantic AI systems to be trained with far less data while still achieving an acceptable degree of accuracy.
#9 Superior customer experience
With its ability to discern user intent, search your entire data estate almost instantaneously, and hold extended conversations with customers to give them the exact answers they need, semantic AI allows you to provide a whole new level of customer experience.
A good example of how this works is ChatGPT, probably the best-known semantic AI implementation in the world today.
This new chatbot has stirred huge interest around the globe. Both professionals and everyday users are excited by its ability to answer a wide range of questions by drawing on information gleaned from millions of sources across the internet.
But ChatGPT also illustrates the current limitations of the technology.
In fact, it doesn't fully understand the broad, real-world implications of the questions it's asked. As a result, ChatGPT sometimes gives answers that, while seemingly plausible, are deceptively inaccurate.
So, it shouldn't yet be trusted to provide definitive answers except in domains where the range of possible responses can be pre-vetted and tightly circumscribed.
Still, this new technology can be immensely useful now and will become more so as it matures. That's why many companies are already rushing to incorporate ChatGPT-like capabilities into their customer service programs.
#10 Greater contextual understanding
Traditional ML is essentially blind to context, which limits the insights it can draw from data.
Semantic AI, instead, by using knowledge graphs and semantically enhanced data, leverages contextual relationships to extract comprehensive insights from its data sources.
The future of semantic AI
Although it is still a young technology, semantic AI is already being used in industries such as banking, finance, journalism, healthcare, and scientific research.
ChatGPT is a good example.
We can expect to see the following trends in the future:
- Semantic data applications will penetrate more industries.
- AI in general will become indispensable for information retrieval and customer service.
- Semantics will be extensively integrated into technologies, tools, and services. That's already happening with, for example, search engines (e.g. Bing chat).
As with any new technology, the best way to get the most out of semantic AI is to work with a partner that has the experience, expertise, and tools to provide effective guidance and support.
Datavid has the right talent to guide you in this new world.
Frequently asked questions
Semantic AI is a branch of artificial intelligence related to natural language processing (NLP) and understanding, which focuses on how computers understand and process human language.
In machine learning, 'semantic' generally refers to the meaning or context of data, rather than its characteristics. Semantic analysis is the process of analysing and interpreting the meaning of data, such as text or images, to extract relevant information and insights.
The semantic web and AI focus on different aspects of intelligent computing. The semantic web is primarily concerned with the organisation and representation of data, while AI focuses on the creation of systems that can reason, learn and make decisions on the basis of such data.
An example of semantic intelligence is a chatbot that is able to understand and respond to natural language queries (e.g., ChatGPT).